Real-Time Machine Learning is Accelerating Growth in Biotech and Pharma
"Real-time analytics is more than just a tool—it’s a paradigm shift," says Dr. Aditya Dodda, an expert in building real-time applications using machine learning. "It allows us to analyze vast and complex data streams in real-time, empowering decision-making at a pace that aligns with the urgency of healthcare innovation."
Trending Photos
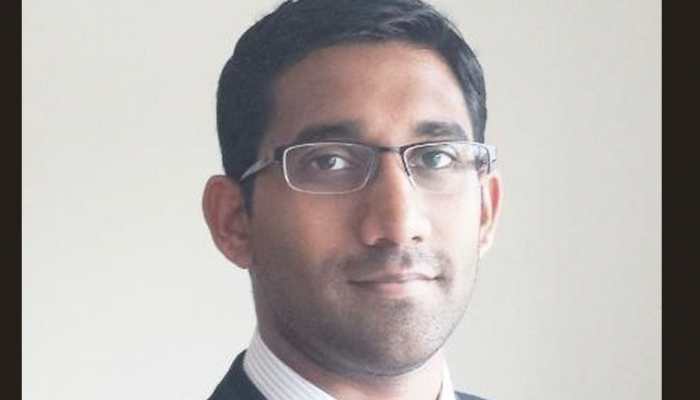)
The biotech and pharmaceutical industries stand at the precipice of an unprecedented transformation. The confluence of machine learning (ML) and numerical optimization is driving the emergence of real-time applications that are revolutionizing drug discovery, development, and manufacturing processes. These technologies are enabling breakthroughs that were once considered the realm of science fiction, accelerating timelines, reducing costs, and improving outcomes across the board.
Real-Time Analytics: Driving Instant Decisions
Real-time analytics is rapidly becoming a cornerstone of the pharmaceutical and biotech sectors. In manufacturing, ML models dynamically adjust parameters to maintain optimal quality and minimize waste. Meanwhile, supply chain optimization algorithms predict and mitigate disruptions, ensuring seamless production.
"Real-time analytics is more than just a tool—it’s a paradigm shift," says Dr. Aditya Dodda, an expert in building real-time applications using machine learning. "It allows us to analyze vast and complex data streams in real-time, empowering decision-making at a pace that aligns with the urgency of healthcare innovation."
From drug discovery to distribution, the ability to act on insights as they occur is transforming how businesses operate and patients are served.
Machine Learning: Revolutionizing Drug Discovery
Machine learning is supercharging the drug discovery pipeline. Traditional methods of identifying promising drug candidates often required years of painstaking effort. Today, real-time ML systems sift through massive chemical libraries in mere hours, identifying viable compounds for further development.
In clinical trials, adaptive algorithms enable real-time adjustments to protocols, optimizing trial designs and enhancing patient outcomes. For instance, anomaly detection models ensure trial data integrity, while predictive analytics help identify potential efficacy issues early.
"ML allows us to think beyond brute force—it's about intelligence and precision," notes Dr. Dodda. "We’re seeing a revolution in how quickly and effectively we can bring new therapies to market."
Numerical Optimization: Ensuring Efficiency and Quality
Numerical optimization is playing a vital role in elevating manufacturing standards in pharma. Advanced optimization techniques help fine-tune production lines in real-time, continuously adjusting key parameters to ensure consistent product quality and efficiency.
Predictive maintenance, another hallmark of real-time optimization, uses data-driven insights to identify equipment issues before they escalate, reducing costly downtime.
"By integrating numerical optimization with machine learning, we’re pushing the boundaries of what's possible in pharmaceutical manufacturing," explains Dr. Dodda. "These advancements are crucial for meeting the growing global demand for high-quality medications."
Edge Computing: Empowering Real-Time Insights
Edge computing is unlocking new opportunities for real-time applications in biotech and healthcare. By processing data locally, edge devices eliminate the delays associated with cloud systems, enabling immediate feedback and adjustments.
In pharmaceutical manufacturing, edge computing ensures rapid decision-making and secure data handling on the production floor. Beyond manufacturing, edge devices like wearable fitness trackers and continuous monitoring systems are revolutionizing patient care, delivering actionable insights directly to users.
"The convergence of edge computing and advanced ML is ushering in a new era of real-time healthcare solutions," says Dr. Dodda. "We can now deploy complex neural networks on edge devices, achieving millisecond-level latency for critical applications like anomaly detection and personalized medicine."
Challenges and Future Prospects
The adoption of real-time systems is not without challenges. Issues like data quality, regulatory compliance, and the scarcity of skilled talent remain key barriers. Yet, the potential benefits far outweigh these obstacles.
Looking ahead, quantum computing combined with machine learning offers a tantalizing glimpse into the future of real-time pharma applications.
"As quantum systems mature, they’ll unlock capabilities for molecular simulations and drug design that are currently beyond our reach," predicts Dr. Dodda. "This integration will further revolutionize healthcare, from personalized medicine to complex diagnostics."
Stay informed on all the latest news, real-time breaking news updates, and follow all the important headlines in india news and world News on Zee News.
Live Tv